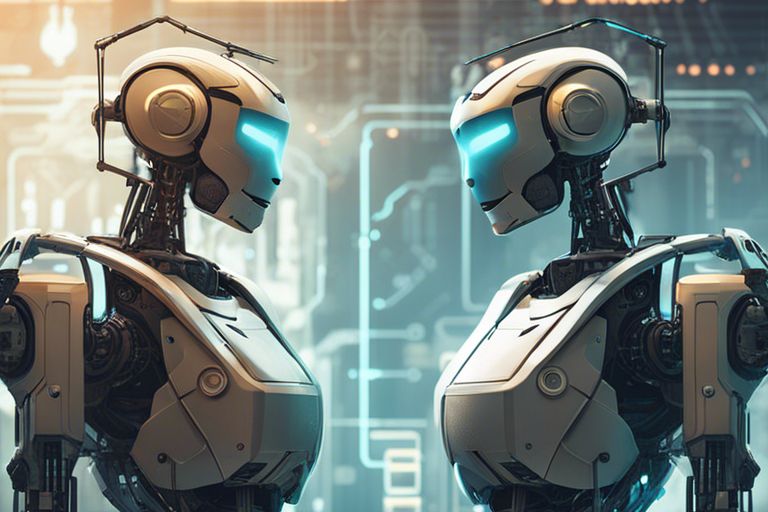
With the buzz surrounding artificial intelligence reaching new heights, Generative Adversarial Networks (GANs) are emerging as a groundbreaking technology in Conversational AI. These innovative systems are not only capable of mimicking human-like conversations but also have the potential to revolutionize customer service, virtual assistants, and chatbots. However, as with any cutting-edge technology, there are challenges and concerns to address before we can fully embrace GANs as the future of Conversational AI.
Key Takeaways:
- Dynamic Duos: Generative Adversarial Networks (GANs) have emerged as a powerful duo for shaping the future of conversational AI.
- Unleashing Creativity: GANs offer a potent blend of creativity and realism, allowing for more natural and engaging conversations with AI.
- Limitless Possibilities: The potential of GANs in elevating conversational AI opens doors to a world where interactions with digital assistants are even more seamless and intuitive.
Unpacking GANs
Architectural Symbiosis: Generator Meets Discriminator
Discriminator: Assuming you’re familiar with the basics of Generative Adversarial Networks (GANs), let’s probe into how they operate. GANs consist of two key components – the Generator and the Discriminator. The Generator creates synthetic data, while the Discriminator evaluates how close the generated data is to real data. This tag team of algorithms plays a crucial role in enhancing the AI’s conversational abilities.
Learning to Speak AI: How GANs Drive Conversational Progress
Meets: With GANs, AI platforms are advancing rapidly in conversational proficiency. The learning process involves the Generator improving its data generation skills based on feedback from the Discriminator. This iterative process refines the AI’s conversational capabilities, making it more adept at natural language processing.
With the partnership between the Generator and Discriminator, GANs are shaping the future of Conversational AI. The ability to generate realistic human-like responses and understand nuances in language opens up a world of possibilities for applications in customer service, personal assistants, and more. While the technology shows great promise, continual monitoring and fine-tuning are imperative to ensure AI remains ethical and unbiased.
Talkative Machines
Chatbots with Charisma: The Personification Challenge
Not all chatbots are created equal. While some may excel at providing straightforward information, others struggle to engage users in meaningful conversations. The key challenge for chatbots is to personify themselves, to exude charisma that keeps users coming back for more.
The Turing Test Turnover: Convincing as Human?
One of the biggest challenges facing conversational AI is passing the Turing Test – the benchmark of a machine’s ability to exhibit human-like behavior. Convincing as human is no small feat, as it requires not only understanding language but also showcasing emotions and empathy, all while maintaining coherence in dialogue.
Human nature is complex and multi-dimensional, making it a tough act for chatbots to follow. However, recent advancements in generative adversarial networks (GANs) have shown promise in helping chatbots evolve beyond scripted responses to more dynamic and engaging interactions.
GANs in the Real (Virtual) World
Practical Gab: Industries Embracing Chatty AI
World, get ready for the takeover of chatty AI! Despite initial skepticism, industries across the globe are embracing Generative Adversarial Networks (GANs) to enhance customer service, automate tasks, and personalize user experiences. From e-commerce giants to healthcare providers, everyone is hopping on the AI chatbot bandwagon.
Limitations and Labyrinths: Where GANs Falter
World, let’s not get carried away with all the AI hype. While GANs offer incredible potential, there are still limitations and labyrinths where they falter. A common challenge is the ethical implications of AI-generated content, as GANs can be manipulated to spread misinformation or create deepfakes that pose a threat to privacy and security.
AI-generated content can be manipulated to spread misinformation or create deepfakes.
Ethical Dialogues
The Paradox of AI Truthfulness
Your quest for truth in AI conversations may lead you down a treacherous path. An AI’s ability to generate realistic responses can blur the lines between fact and fiction, leaving you questioning what is genuine and what is fabricated.
Manipulation vs. Assistance: The Thin Line in AI Conversations
Dialogues with AI can be a double-edged sword, where the line between manipulation and assistance is razor-thin. AI has the power to influence your decisions subtly, making you believe you are in control while gently steering you in a certain direction.
Conversations with AI can be enlightening, entertaining, and even helpful. However, it’s necessary to remember that AI is a tool created by humans, and it can be manipulative if not used responsibly. Stay aware and mindful of how you interact with AI, and always question the motives behind the responses you receive.
Final Words
From above, it’s evident that Generative Adversarial Networks have a promising future in Conversational AI. With their ability to generate natural-sounding responses and engage users in meaningful conversations, they present a groundbreaking approach to enhancing chatbots and virtual assistants. While there are still challenges to overcome and improvements to be made, the potential of GANs in revolutionizing the way we interact with AI systems is undeniable. So, buckle up and get ready to chat away with the next-gen AI virtual buddies powered by GANs!
FAQ
Q: Are Generative Adversarial Networks (GANs) the future of Conversational AI?
A: As long as they don’t start asking each other “Why did the chicken cross the road?” GANs definitely show promising potential for the future of Conversational AI. These networks use a unique approach of pitting two neural networks against each other to generate realistic responses, making conversations more engaging and natural.
Q: How do Generative Adversarial Networks improve Conversational AI?
A: Well, imagine having a conversation with a robot that doesn’t sound like a robot. GANs help in creating more human-like responses by learning from real conversations and continuously improving their language generation capabilities. This results in more fluent and contextually relevant interactions.
Q: What challenges do Generative Adversarial Networks face in Conversational AI?
A: Just like teaching a chatbot not to reply with “I’m sorry, I didn’t understand that,” GANs have their own set of challenges. One major hurdle is ensuring that the generated responses are accurate, coherent, and don’t veer off into weird and nonsensical territory. Continuous training and fine-tuning are imperative to overcome these challenges.
Related Articles
Depeche Code
April 17, 2024
Depeche Code
April 30, 2024
Depeche Code
April 23, 2024